What Can You Foresee With Predictive Modeling?
Today, no business develops blindly. Instead, companies draw up development plans where they set goals and define ways to achieve them. Such plans are based on previous experience. They use historical data to suggest scenarios for business growth or decline. To make them as realistic as possible, companies rely on predictive analytics technologies. With their help, they create forecasting models that describe the future business state in the most accurate way.
Adopting predictive analytics is a good idea for enterprises aiming to enhance business productivity. However, the companies should be ready to face some challenges on the way to implementation. The nature of those challenges – and how to overcome them – is our topic for today.
Challenges in predictive analytics
Supervised learning. Artificial intelligence (AI) is evolving every day, but it still requires human supervision. Most AI models today are trained on data that is manually labeled by humans. This is quite a costly affair with a high probability of error. To solve the problem, companies should look towards unsupervised learning. There are two ways to set it up:
through the model learning during a process of trial and error
by comparing the concept understanding of two competing models
Insufficient data. To teach a predicting algorithm to make the right decisions, you need to feed it with big data, which is not always possible to obtain. Imagine, for example, machine learning (ML) prediction in healthcare. AI should consider many factors in various combinations to make a correct diagnosis. Given this, the one-shot learning technique should gain higher adaptability. It allows AI to draw valid conclusions after seeing a single sample – for example, a picture of a patient with symptoms of chickenpox or measles.
Inability to explain results. ML engineers cannot always understand why a predictive model made a particular conclusion. However, model transparency is required because model decisions affect business-important actions, and sometimes lives. There are two ways to fix the problem:
analyze data the model uses when making a decision
analyze data the model prioritizes when making a decision (for example, product price increase when predicting income)
Inflexibility in knowledge application. The predictive capabilities of machines are limited when applied to unfamiliar use cases, even when those cases are similar to those on which the model was trained. In other words, machines cannot apply their knowledge in different circumstances. Instead, they need to be retrained for each new case, which is time and resource-consuming. To cut costs and make ML models reusable, developers may apply the transfer learning technique. It enables them to teach a model on a given use case and then quickly transfer this knowledge to similar, but not identical, cases.
Bias in data and algorithms. A model learning under human supervision will make the same mistakes as its teacher. The bias is especially dangerous for predictive analysis in medicine, financial forecasts, and economic predictive modeling. Moreover, it tends to be reproduced in similar cases. So, you need to look for ways to provide the most objective data. One way to ensure objectivity is to adopt unsupervised learning and minimize human participation in model education.
Benefits of predictive modeling
Organizations that use predictive analytics are one step ahead of their competitors. Through data prediction, companies gain futuristic insights helping them make informed decisions and tune their businesses for economic growth. Here are just a few benefits smart forecasting brings:
Demand prediction. Predictive analytics techniques help contemplate future demand, considering current sales rates, market conditions, and customer behavior patterns.
Workforce planning. Having informed insights, organizations can estimate the salary budget and start looking for the relevant specialists.
Competitors analysis. Predictive reporting is able to forecast in which direction competitors will develop and their prospective profit.
Financial risks identification. Predictive analytics will show the main risks for future activity by considering the current business state and external factors.
Customer churn prediction. By understanding the reasons for possible customer churn, companies can take steps to alleviate any such loss.
Top-5 use cases of predictive analytics in enterprises
ML algorithms process vast amounts of data and deliver valuable results for any business area. The following are the most common examples of applying predictive analytics in various industries.
Healthcare
There are various examples and application types of predictive modeling in healthcare. First of all, it serves for making a correct diagnosis. ML algorithms consider multiple factors influencing the patient, such as environment, social status, living habits, past illnesses, etc. This helps identify the roots of disease, make a correct diagnosis, and assign a proper treatment. Besides, the doctor can foresee possible future ailments and take measures beforehand.
Another healthcare use case is connected with analyzing treatment efficiency. For example, hospitals may collect data on the treatment of the same diseases by different doctors in different departments. Thus, they can detect the most efficient ways to treat the patients and share their findings for the benefit of society.
Ysbyty Gwynedd use case: early symptoms detection
Ysbyty Gwynedd, the General Hospital in Bangor, uses Philips IntelliVue Guardian Software to reduce manual health audits. Thanks to Early Warning Score, the software alerts nurses when a patient’s condition is about to worsen so they can take timely measures and reduce serious events.
Supply chain
Predictive modeling can calculate the delivery time for supply chain companies. It makes predictions based on various factors, such as traffic jams, road quality, vehicle speed, transport conditions, etc. Companies which become aware of any factor delaying on-time delivery, can make timely adjustments and ensure that the delivery process continues to run smoothly.
In addition, intelligent algorithms can simulate various scenarios based on risk factors. This allows companies to be very agile in their supply chain strategy and choose the most efficient delivery method in a given situation. For example, they can postpone the delivery or choose another road if the weather conditions are poor.
Erbis use case: real-time tracking
Erbis developed a supply chain management system for a San Francisco-based company. Thanks to our solution, the client has complete visibility for global supply chains with real-time insights about delivery costs, transactions, and other relevant supply chain information.
Real estate
One of the primary brokerage services is real estate asset evaluation. With predictive analytics, real estate agents can provide more accurate calculations and prove the non-bias of their assessment. Such ML-based estimates can enhance the clients’ opinions of the professionalism of agents, and strengthen brand confidence. Thus, an edge can be gained over competitors by showing the adoption of new technologies and high customer focus.
Another use case of predictive analytics in real estate is matching ready-and-able buyers with potential sellers. The latter may not be ready to offer their homes for sale until they see buyers with money. In this way, innovative technologies can help them make an informed decision and negotiate a good deal.
Zillow use case: holistic analytics
Zillow, one of the largest real estate platforms, uses intelligent analytics for multiple cases. Smart technologies help them form prices, advise the best time to list, recommend homes based on user preferences, and do many more things, which you can see below:
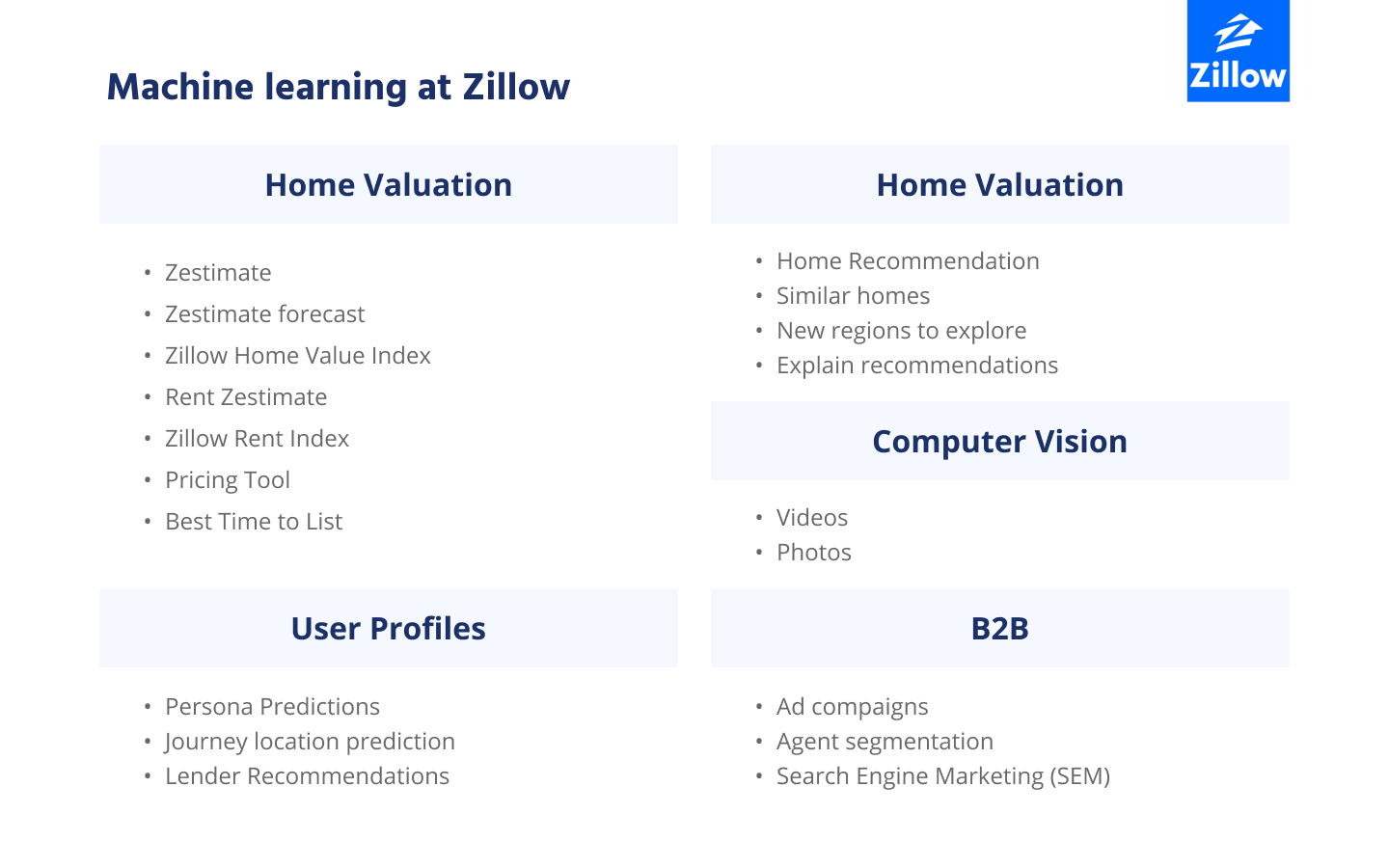
Retail
In a highly competitive environment, retailers must put effort into engaging customers. They should react to changes in buyers’ behavioral patterns, preventing them from being lost to competitors. Predictive analytics techniques serve well for analyzing customers’ moods. They predict possible customer churn, letting organizations take timely measures to prevent this. For example, having noticed a decrease in customer activity, the seller may offer a personal discount or bonuses for the next purchase. In addition, analytics can help segment customers and identify those who are willing to spend more. For these buyers, companies can create individual marketing campaigns that will encourage them to make more purchases.
Carrefour use case: inventory management
Carrefour, a French multinational retail corporation, uses AI-powered analytics to predict demand and plan supply. According to the predicted demand figures, they stock up the goods, avoiding shortages or overstock of particular products.
Insurance
Global fraud detection is growing from year to year. Unfortunately, insurance is one of the most vulnerable industries. Criminals are continually devising schemes to defraud companies. Predictive analytics models can help insurers stay safe. They study previous fraud cases and quickly warn owners if similar schemes are detected. Besides, they monitor all system users and alert companies if suspicious actions are noticed. Thus, real-time predictive analytics help companies protect against upcoming attacks and reduce the likelihood of suffering losses.
Lemonade use case: claims processing
Lemonade, an international insurance company, uses chatbots to handle initial inquiries. Smart assistants check customer history, analyze their applications, and alert a company about a possible fraud attempt. Thus, they will filter out criminals during the first contact, not allowing them to proceed.
Conclusion
Predictive modeling gives companies insights into business development and industry trends. Intelligent algorithms learn from vast amounts of information and apply this knowledge to predict human diseases, financial risks, product prices, customer churn, possible fraud, etc. Organizations that adopt forecasting models have a distinct advantage over their competitors. Knowing what to expect in the future, they can revamp business activity and increase chances for profit growth.